Title: AI Models Get Smarter by Asking Users More Questions, Research Shows
Subtitle: A team of researchers led by Anthropic has developed a method called “generative active task elicitation (GATE)” to help AI models understand user preferences and provide more accurate responses.
VentureBeat presents: AI Unleashed – An exclusive executive event for enterprise data leaders. Network and learn with industry peers. Learn More
In a breakthrough study published by researchers from Anthropic and leading institutions such as MIT and Stanford, a new method called “generative active task elicitation (GATE)” has been developed to enhance AI models’ ability to understand user preferences. This groundbreaking approach involves AI models asking users more questions to determine their true preferences when interacting with the models.
Anthropic researcher Alex Tamkin, along with colleagues Belinda Z. Li, Jacob Andreas, and Noah Goodman, presented their findings in a research paper titled “Eliciting Human Preferences With Language Models.” The researchers aim to utilize large language models (LLMs) to convert human preferences into automated decision-making systems.
The GATE method allows AI models to analyze and generate text while incorporating user answers to shape future interactions. By inferring the meaning behind the user’s responses based on their relationship to other concepts within the LLM’s database, the AI model can better understand what the user wants.
The GATE method encompasses three different approaches:
1. Generative active learning: The AI model produces examples of potential responses and asks users if they find them desirable. The model then adjusts its output based on the user’s feedback.
2. Yes/no question generation: The model asks binary yes or no questions to gauge the user’s preferences. It takes into account the user’s answers and avoids providing information associated with a negative response.
3. Open-ended questions: The model seeks to obtain broad and abstract knowledge from the user, asking questions about their hobbies or activities and capturing their motivations.
The researchers tested the GATE method in three domains: content recommendation, moral reasoning, and email validation. By fine-tuning an Anthropic rival’s AI model with GATE and gathering responses from 388 paid participants, the researchers found that GATE consistently outperformed baseline models with comparable or even less mental effort from users.
Specifically, the fine-tuned AI model exhibited a better understanding of each user’s individual preferences, as measured subjectively. This improvement, even at a small scale, has significant implications for enterprise software developers looking to enhance user experiences with AI-powered chatbots.
By implementing the GATE method, developers can save valuable time by bypassing extensive training on large datasets. Instead, they can tailor their AI models to directly cater to individual customer preferences and deliver engaging and helpful experiences.
As the GATE method gains popularity, users may notice their AI chatbots asking more questions about their preferences. This shift represents an exciting leap forward in the field of AI, as models become more adept at understanding and meeting users’ needs.
VentureBeat’s mission is to serve as a digital platform for technical decision-makers to access knowledge about transformative enterprise technology. Discover our Briefings.
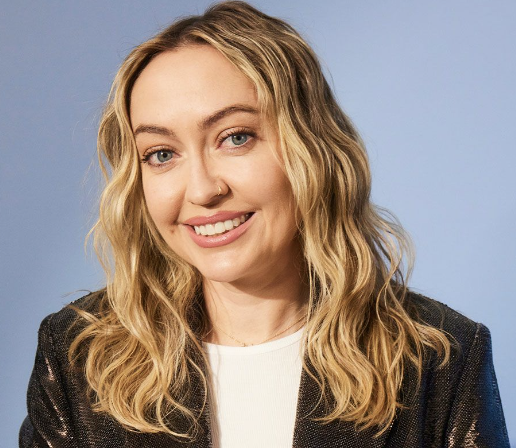
I’m a highly experienced and respected author in the field of cryptocurrency. I have been writing about Bitcoin, Ethereum, Litecoin and other digital currencies for over 5 years which is widely regarded as one of the most knowledgeable and reliable sources of information in this area.